Introduction: The Convergence of Cutting-Edge Technologies
In the rapidly evolving world of investing, technology continues to play an increasingly significant role. Artificial Intelligence (AI), Machine Learning (ML), and Quantum Computing are three cutting-edge technologies that have the potential to revolutionize the investing landscape. By combining AI, ML, and quantum computing for investing, investors can make more informed, strategic, and efficient decisions. These advanced technologies can analyze vast amounts of data, identify patterns, adapt to changing market conditions, and solve complex problems at unprecedented speeds.
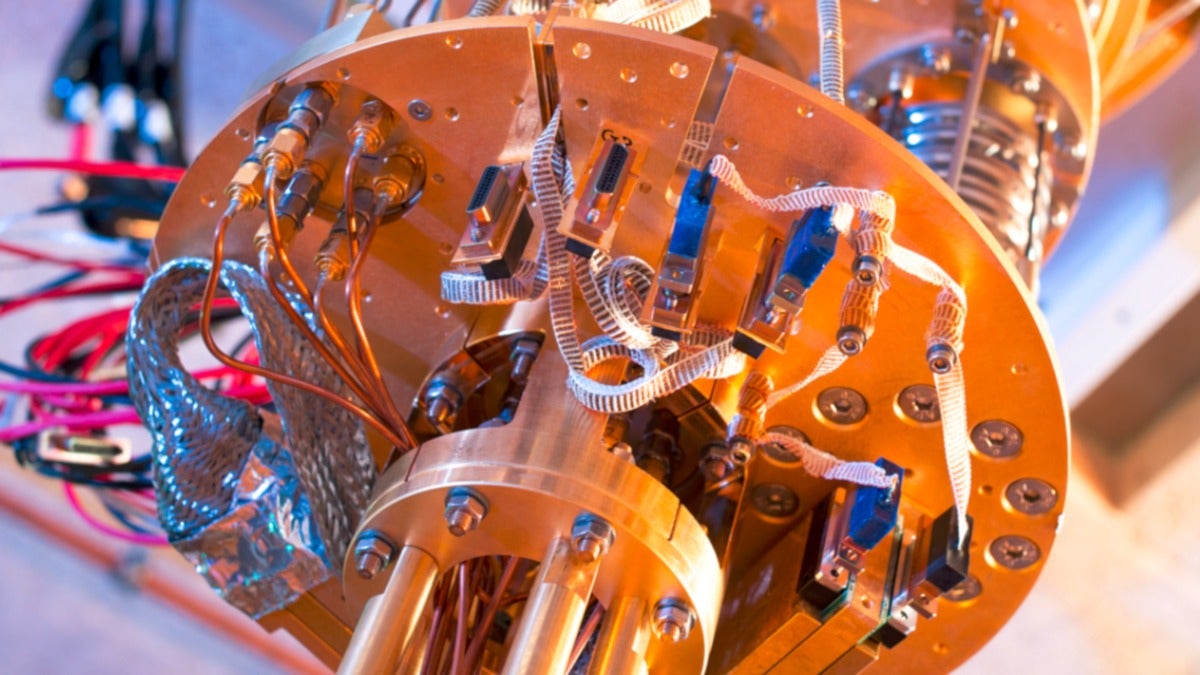
Artificial Intelligence (AI) in Investing: Unlocking Efficiency and Insights
Artificial Intelligence (AI) has emerged as a powerful tool in the investing world, offering the ability to analyze vast amounts of data, identify patterns, and make predictions. By automating time-consuming tasks and providing real-time insights, AI-driven platforms and tools help investors make more informed and strategic decisions. Some examples of AI applications in investing include robo-advisors, natural language processing (NLP) for sentiment analysis, and predictive analytics for risk assessment.
Robo-advisors, such as Betterment and Wealthfront, leverage AI algorithms to create and manage investment portfolios tailored to individual investors’ goals, risk tolerance, and time horizon. These platforms offer a cost-effective and efficient alternative to traditional human advisors, making professional investment management accessible to a broader audience.
Natural Language Processing (NLP), a subfield of AI, enables computers to understand, interpret, and generate human language. In investing, NLP can be used to analyze news articles, social media posts, and other text-based data to gauge market sentiment and identify potential investment opportunities. For instance, AI-powered platforms like Amenity Analytics and Aylien use NLP to analyze unstructured data and extract actionable insights for investors.
Predictive analytics, another AI application, involves using historical data and machine learning algorithms to forecast future trends and events. In the investing context, predictive analytics can help investors assess risk, identify undervalued assets, and anticipate market movements. Companies like Kavout and Numerai use AI and machine learning to analyze vast datasets and generate predictive models for their users.
Machine Learning (ML) in Investing: Adaptive and Data-Driven Decisions
Machine Learning (ML), a subset of Artificial Intelligence (AI), has gained significant traction in the investing landscape due to its ability to learn from data, improve over time, and adapt to changing market conditions. ML algorithms analyze patterns, identify trends, and make predictions based on historical data, enabling investors to make more informed and strategic decisions.
ML applications in investing can be broadly categorized into two areas: investment strategies and portfolio management. In investment strategies, ML algorithms can analyze vast datasets, including financial statements, economic indicators, and news articles, to identify potential investment opportunities. For instance, ML models can be trained to predict stock prices, analyze market sentiment, or identify undervalued assets. Companies like Sentient Investment Management and Aidyia Limited use ML algorithms to generate trading signals and execute automated trades based on these insights.
In portfolio management, ML can help optimize asset allocation, manage risk, and improve overall investment performance. ML algorithms can analyze an investor’s risk tolerance, investment goals, and time horizon to create a customized portfolio tailored to their specific needs. Furthermore, ML can be used to monitor portfolio performance, rebalance assets, and adjust investment strategies in real-time, ensuring that the portfolio remains aligned with the investor’s objectives.
One example of ML in portfolio management is the work of Bridgewater Associates, the world’s largest hedge fund. Bridgewater has developed an AI-powered system called “Pure Alpha,” which uses ML algorithms to analyze economic trends, market data, and geopolitical events to make informed investment decisions. Pure Alpha has reportedly generated significant returns for Bridgewater’s clients, demonstrating the potential of ML in portfolio management.
Despite the benefits of ML in investing, there are also potential challenges and limitations. ML models can be prone to overfitting, where the model becomes too specialized to the training data and performs poorly on new, unseen data. Additionally, ML models can be opaque and difficult to interpret, making it challenging to understand the reasoning behind their predictions. To address these challenges, it is essential to employ best practices in ML model development, including rigorous testing, validation, and interpretation.
Quantum Computing in Investing: A New Era of Computational Power
Quantum computing, a cutting-edge technology, has the potential to revolutionize the investing industry by solving complex problems and processing massive datasets at unprecedented speeds. Unlike classical computers, which use bits to represent information as either 0 or 1, quantum computers use quantum bits, or qubits, which can exist in multiple states simultaneously, enabling them to perform multiple calculations simultaneously.
The power of quantum computing lies in its ability to perform complex calculations and simulations that are beyond the capabilities of classical computers. In the investing world, quantum computing can be used to analyze vast amounts of data, identify patterns, and make predictions with unprecedented accuracy. For instance, quantum computers can be used to optimize trading strategies, manage risk, and improve overall investment performance.
One example of quantum computing in investing is the work of Cambridge Quantum Computing (CQC), a UK-based company that has developed a quantum-enhanced portfolio optimization tool. This tool uses quantum algorithms to optimize investment portfolios, taking into account factors such as risk tolerance, investment goals, and market conditions. By using quantum computing, CQC’s tool can perform complex calculations and simulations in a fraction of the time it would take a classical computer, enabling investors to make more informed and strategic decisions.
Despite the potential benefits of quantum computing in investing, there are also potential challenges and limitations. Quantum computers are still in the early stages of development, and there are significant technical challenges to overcome before they can be widely adopted. Additionally, quantum computers require specialized hardware and software, which can be expensive and difficult to obtain. To address these challenges, it is essential to invest in research and development, build partnerships with technology companies, and develop a skilled workforce capable of working with quantum computing technology.
Furthermore, the integration of quantum computing in investing raises ethical and regulatory considerations. Quantum computing has the potential to exacerbate existing inequalities in the investing world, as those who have access to quantum computing technology may have an unfair advantage over those who do not. To address these concerns, it is essential to develop regulatory frameworks that ensure transparency, fairness, and compliance in the use of quantum computing technology in investing.
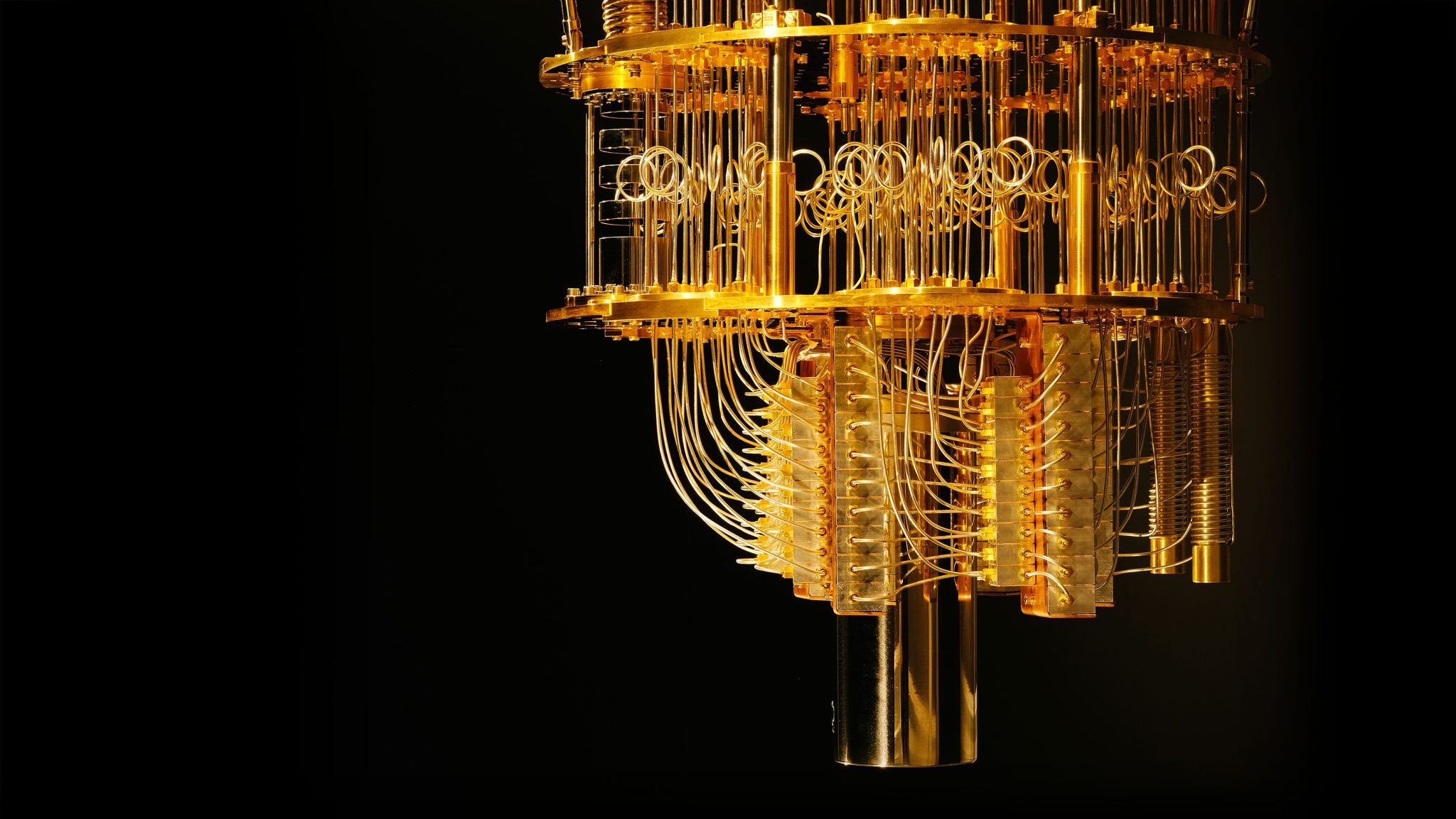
How to Combine AI, ML, and Quantum Computing for Investing
Combining AI, ML, and quantum computing for investing is a powerful strategy that can lead to more informed and strategic investment decisions. By integrating these cutting-edge technologies, investors can analyze vast amounts of data, identify patterns, and make predictions with unprecedented accuracy. However, there are several steps and considerations to keep in mind when integrating these technologies in an investment strategy.
The first step is to identify the specific investment problem or opportunity that you want to address. This will help you determine which technology or combination of technologies is best suited for your needs. For example, if you are looking to optimize a trading strategy, AI and ML algorithms can be used to analyze historical data, identify patterns, and make predictions about future market movements. On the other hand, if you are looking to solve complex optimization problems, such as portfolio optimization, quantum computing can be used to perform complex calculations and simulations that are beyond the capabilities of classical computers.
Once you have identified the specific investment problem or opportunity, the next step is to select the appropriate technology or combination of technologies. This will require a thorough understanding of the strengths and limitations of each technology. For example, AI and ML algorithms are well-suited for tasks that involve pattern recognition, prediction, and decision-making, while quantum computing is well-suited for tasks that involve complex optimization problems and large-scale simulations.
The third step is to develop a strategy for integrating the selected technology or technologies into your investment process. This will require a thorough understanding of the data sources, algorithms, and infrastructure required for each technology. For example, if you are using AI and ML algorithms, you will need to identify the relevant data sources, develop the algorithms, and build the infrastructure required for data processing and analysis. Similarly, if you are using quantum computing, you will need to identify the relevant quantum algorithms, build the quantum hardware and software infrastructure, and integrate it with your existing investment process.
When integrating AI, ML, and quantum computing in an investment strategy, there are several potential challenges and benefits to keep in mind. One potential challenge is the complexity and cost of integrating these cutting-edge technologies. This will require significant investment in research and development, as well as the acquisition of specialized hardware and software. Additionally, there are potential ethical and regulatory challenges associated with the use of these technologies in investing, which will require careful consideration and management.
Despite these challenges, there are also several potential benefits to combining AI, ML, and quantum computing in an investment strategy. For example, these technologies can enable investors to analyze vast amounts of data, identify patterns, and make predictions with unprecedented accuracy. Additionally, they can enable investors to solve complex optimization problems, such as portfolio optimization, that are beyond the capabilities of classical computers. Furthermore, they can enable investors to adapt to changing market conditions and make data-driven decisions in real-time.
There are several real-world examples of successful implementations of AI, ML, and quantum computing in investing. For example, BlackRock, the world’s largest asset manager, has developed an AI-driven investment platform that uses machine learning algorithms to analyze vast amounts of data and make predictions about future market movements. Similarly, IBM has developed a quantum-enhanced portfolio optimization tool that uses quantum algorithms to optimize investment portfolios, taking into account factors such as risk tolerance, investment goals, and market conditions.
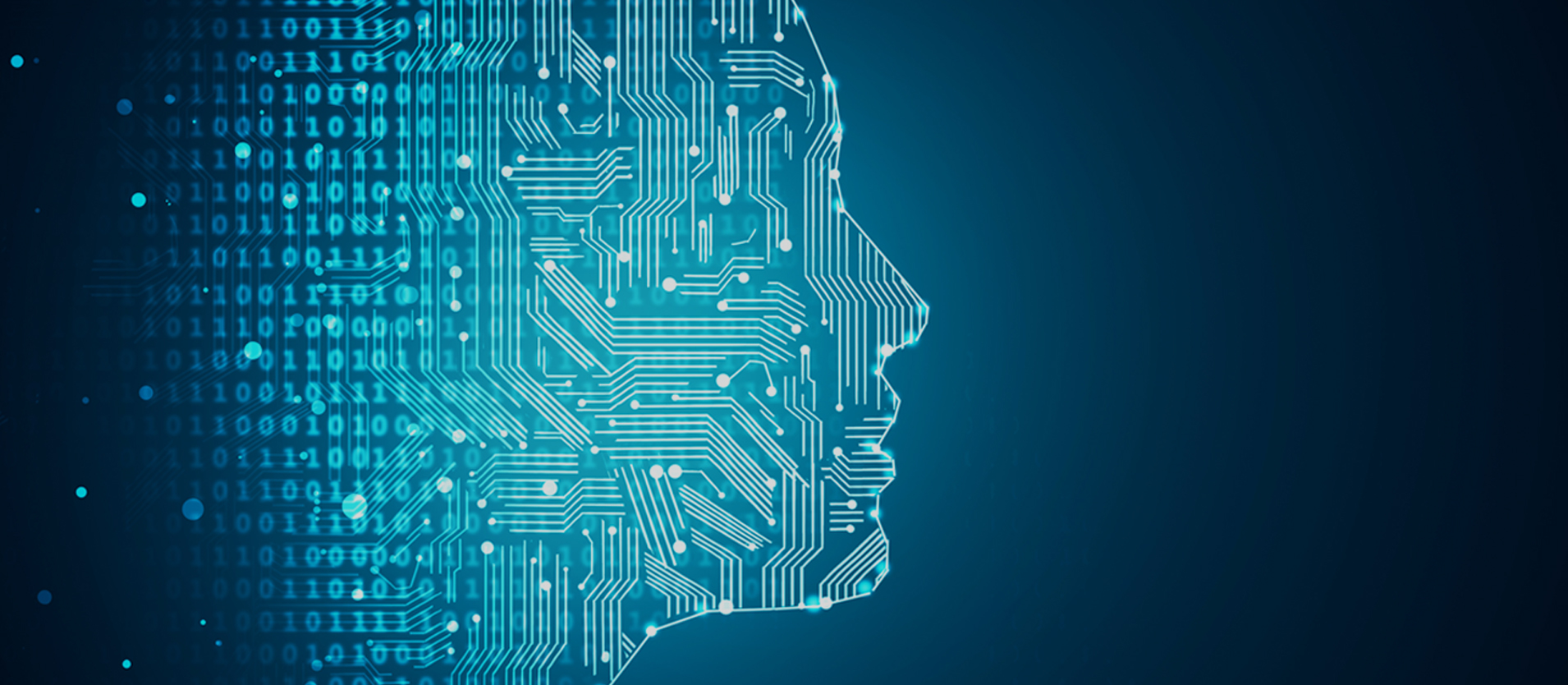
Ethical Considerations and Regulatory Challenges
As with any emerging technology, combining AI, ML, and quantum computing for investing raises ethical implications and regulatory challenges. It is crucial to address these issues to ensure transparency, fairness, and compliance in the investment process.
One ethical concern is the potential for bias in AI and ML algorithms. These algorithms are trained on historical data, which may contain biases that can be perpetuated in the investment decision-making process. To mitigate this risk, it is essential to use diverse and representative data sets and regularly audit the algorithms for bias.
Another ethical consideration is the potential for AI and ML algorithms to replace human investment professionals, leading to job displacement. To address this concern, it is important to view AI and ML as tools that can augment human decision-making rather than replace it. By combining the strengths of humans and machines, investors can make more informed and strategic investment decisions.
Regulatory challenges also arise when combining AI, ML, and quantum computing for investing. These technologies operate in a rapidly changing regulatory environment, with new rules and regulations being introduced regularly. To ensure compliance, it is essential to stay up-to-date with regulatory developments and work with regulators to develop appropriate guidelines and frameworks.
One potential solution to these challenges is to establish a regulatory sandbox, which allows companies to test new technologies in a controlled environment with regulatory oversight. This approach can help ensure that AI, ML, and quantum computing are used ethically and responsibly in the investment process while promoting innovation and growth.
Another best practice is to ensure transparency in the investment decision-making process. This can be achieved by providing clear and concise explanations of the algorithms and data sets used in the investment process and making this information available to investors and regulators.
By addressing these ethical implications and regulatory challenges, investors can harness the power of AI, ML, and quantum computing while ensuring transparency, fairness, and compliance in the investment process.

The Future of Investing: AI, ML, and Quantum Computing
Combining AI, ML, and quantum computing for investing holds the potential to revolutionize the investing landscape, creating new opportunities for investors and businesses alike. By harnessing the power of these cutting-edge technologies, investors can make more informed and strategic investment decisions, adapt to changing market conditions, and solve complex problems at unprecedented speeds.
One of the most significant implications of combining these technologies is the ability to process and analyze massive amounts of data in real-time. With AI and ML algorithms, investors can identify patterns, make predictions, and adapt to changing market conditions more efficiently than ever before. Quantum computing takes this a step further by solving complex problems and processing massive datasets at unprecedented speeds.
In the long term, combining AI, ML, and quantum computing for investing could lead to the development of new investment strategies and products that are more adaptive, personalized, and efficient. For example, investors could use AI and ML algorithms to create dynamic portfolios that automatically adjust to changing market conditions, or to develop new investment products that are tailored to individual investor preferences and risk profiles.
Moreover, combining these technologies could help investors make better-informed decisions by providing access to real-time market data, news, and social media sentiment analysis. By analyzing this data in real-time, investors can gain a better understanding of market trends, identify potential risks and opportunities, and make more informed investment decisions.
However, combining AI, ML, and quantum computing for investing also raises ethical implications and regulatory challenges. It is crucial to address these issues to ensure transparency, fairness, and compliance in the investment process. This can be achieved by establishing regulatory sandboxes, ensuring transparency in the investment decision-making process, and regularly auditing AI and ML algorithms for bias.
In conclusion, combining AI, ML, and quantum computing for investing holds the potential to revolutionize the investing landscape, creating new opportunities for investors and businesses alike. By harnessing the power of these cutting-edge technologies, investors can make more informed and strategic investment decisions, adapt to changing market conditions, and solve complex problems at unprecedented speeds. However, it is essential to address the ethical implications and regulatory challenges associated with these technologies to ensure transparency, fairness, and compliance in the investment process.
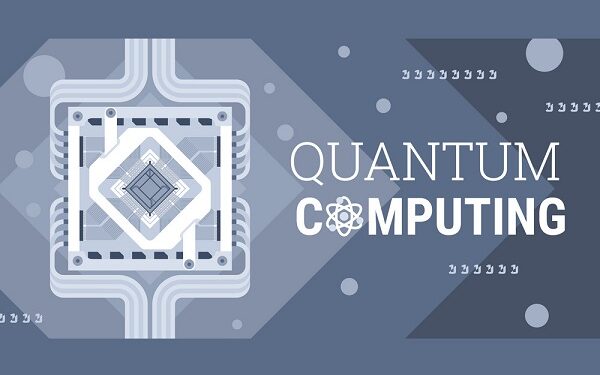
Conclusion: Embracing the AI, ML, and Quantum Computing Revolution
In conclusion, combining AI, ML, and quantum computing for investing holds the potential to revolutionize the investing landscape, creating new opportunities for investors and businesses alike. By harnessing the power of these cutting-edge technologies, investors can make more informed and strategic investment decisions, adapt to changing market conditions, and solve complex problems at unprecedented speeds.
To get started with integrating AI, ML, and quantum computing in your investment strategy, consider the following actionable tips:
- Stay informed about the latest developments in AI, ML, and quantum computing by following industry news and publications.
- Explore AI-driven investment platforms and tools that can help you analyze vast amounts of data, identify patterns, and make predictions.
- Consider working with a financial advisor or investment manager who has experience in integrating AI, ML, and quantum computing in investment strategies.
- Ensure transparency and fairness in your investment decision-making process by establishing clear guidelines and regularly auditing AI and ML algorithms for bias.
- Stay up-to-date with regulatory developments and ensure compliance with relevant regulations and best practices.
By embracing the AI, ML, and quantum computing revolution, investors can gain a competitive edge in the investing world and unlock new opportunities for growth and success. With the right approach and resources, you can harness the power of these cutting-edge technologies to make more informed and strategic investment decisions, adapt to changing market conditions, and achieve your financial goals.
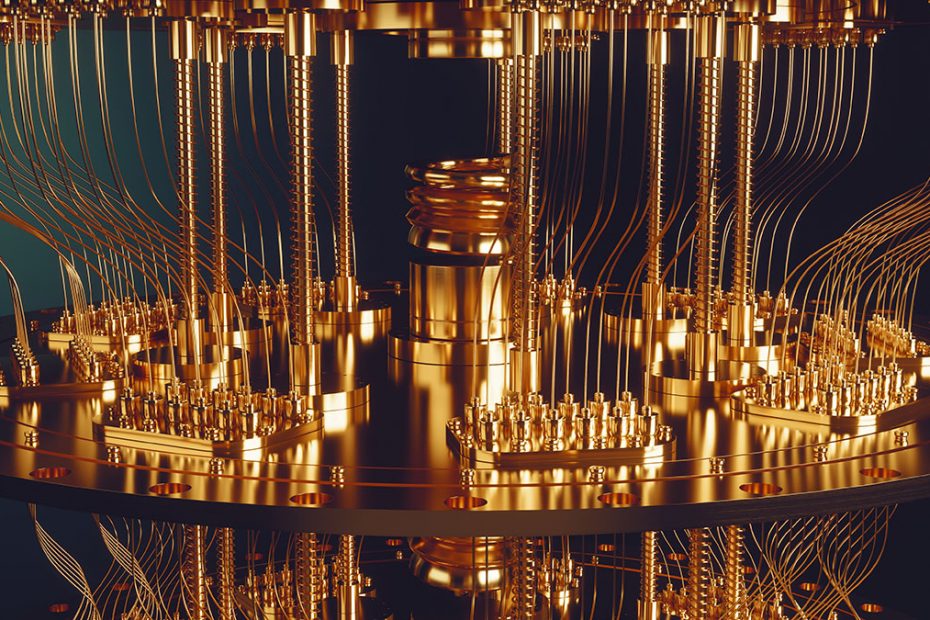