Understanding the Fundamentals of Research Methodologies
In the realm of research, the design of a study is crucial in drawing meaningful conclusions. A well-crafted research design enables researchers to collect and analyze data effectively, ultimately leading to reliable and generalizable findings. When it comes to research methodologies, two primary approaches stand out: cross-sectional and time series. Understanding the differences between these two approaches is essential in choosing the right methodology for a study. The choice of research design can significantly impact the validity and reliability of the results, making it a critical decision in the research process.
The cross-sectional vs time series debate has been a longstanding one in the research community. While both approaches have their strengths and weaknesses, they are suited for different research questions and goals. Cross-sectional research, for instance, is ideal for studies that aim to capture a snapshot of a phenomenon at a particular point in time. On the other hand, time series research is better suited for studies that seek to analyze trends and patterns over time. By understanding the characteristics, advantages, and limitations of each approach, researchers can make informed decisions about the most appropriate design for their study.
In this article, we will delve into the world of research design, exploring the fundamentals of cross-sectional and time series research. We will examine the benefits and drawbacks of each approach, discuss how to choose the right research design for a study, and highlight the practical applications of both methodologies in various fields. By the end of this article, readers will have a comprehensive understanding of the cross-sectional vs time series debate and be equipped with the knowledge to make informed decisions about their research design.
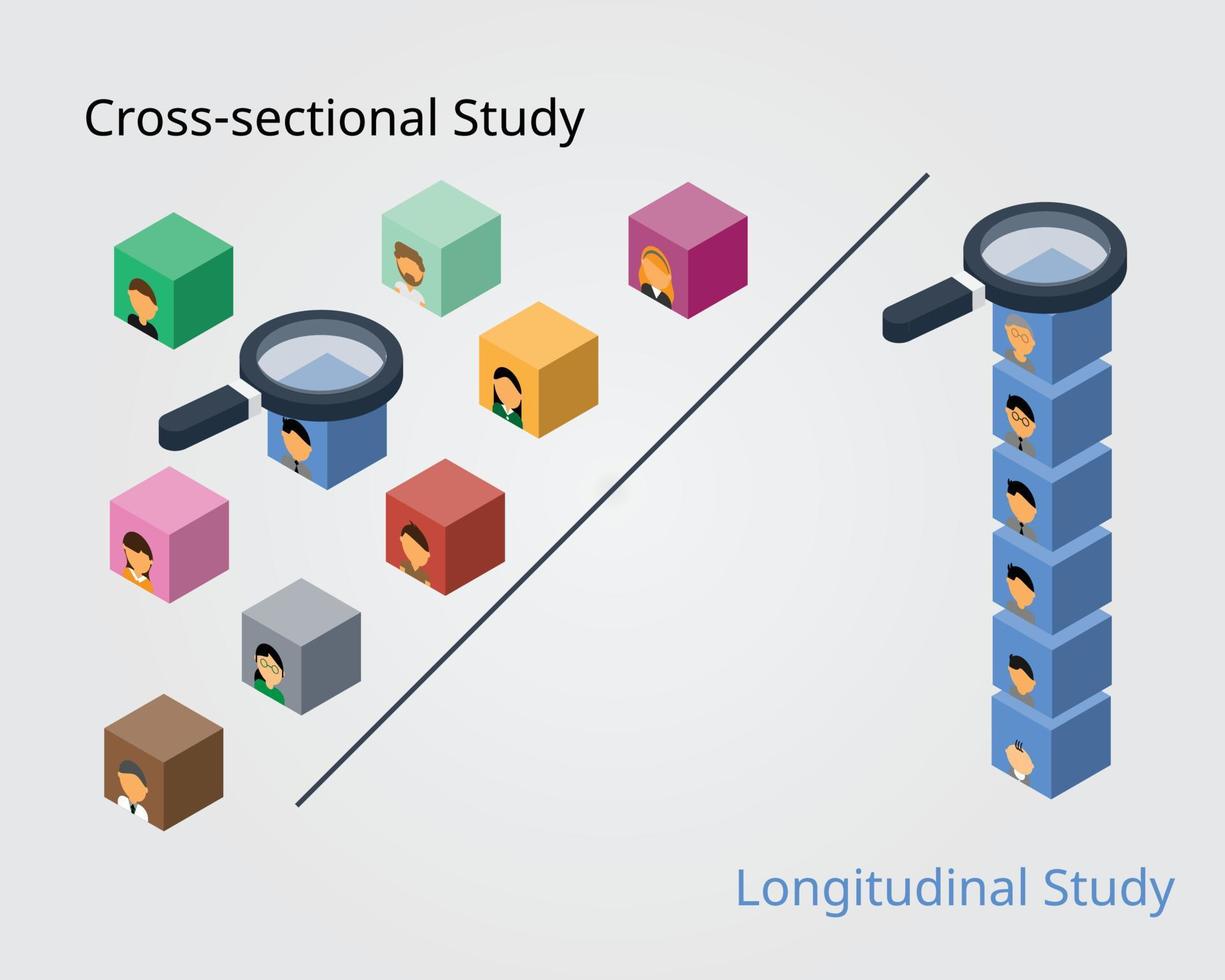
What is Cross-Sectional Research? Unpacking its Advantages and Limitations
Cross-sectional research is a type of study that involves collecting data from a sample of participants at a single point in time. This approach is often used to examine the relationships between variables, identify patterns, and make predictions. One of the primary advantages of cross-sectional research is its cost-effectiveness and ease of data collection. Researchers can quickly gather data from a large sample of participants, making it an ideal approach for studies with limited resources.
Another benefit of cross-sectional research is its ability to provide a snapshot of a phenomenon at a particular point in time. This can be particularly useful in studies that aim to understand the prevalence of a particular condition or behavior. For instance, a cross-sectional study can be used to examine the prevalence of a disease in a population, providing valuable insights for healthcare professionals and policymakers.
However, cross-sectional research also has its limitations. One of the primary drawbacks is the lack of longitudinal data, which can make it difficult to establish cause-and-effect relationships between variables. Additionally, cross-sectional research is susceptible to potential biases, such as sampling biases and measurement errors. Researchers must be aware of these limitations and take steps to mitigate them in order to ensure the validity and reliability of their findings.
In the context of the cross-sectional vs time series debate, cross-sectional research is often preferred when researchers need to answer questions about the relationships between variables at a single point in time. However, when researchers need to examine trends and patterns over time, time series research may be a more suitable approach. By understanding the advantages and limitations of cross-sectional research, researchers can make informed decisions about the most appropriate design for their study.
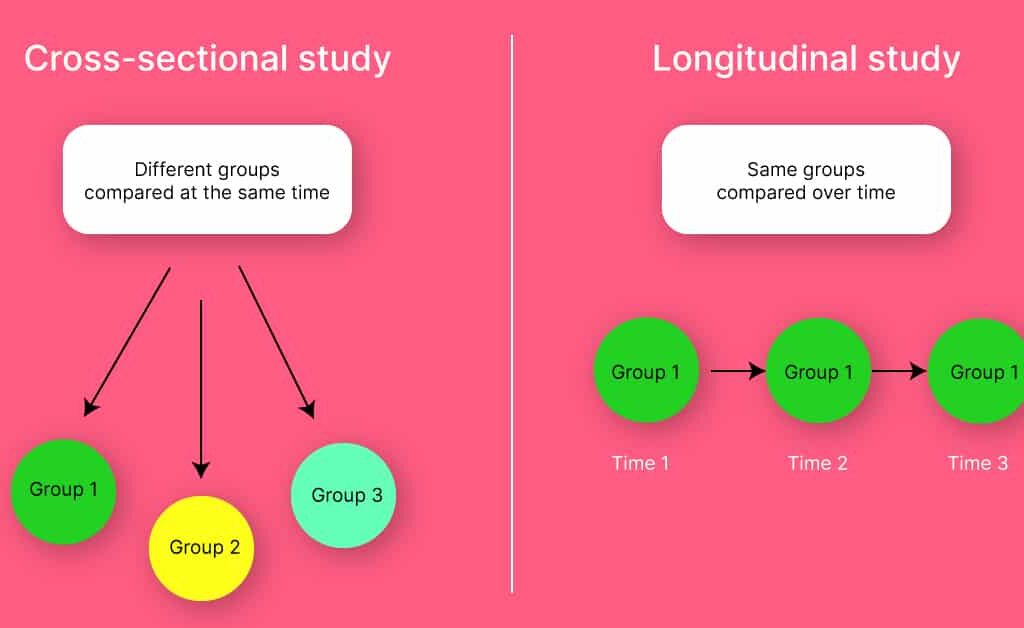
Time Series Research: A Deep Dive into its Applications and Drawbacks
Time series research is a type of study that involves collecting and analyzing data over a prolonged period. This approach is particularly useful for examining trends, patterns, and relationships between variables over time. One of the primary benefits of time series research is its ability to identify causal relationships between variables, allowing researchers to make predictions and forecasts.
Time series research is also ideal for studying phenomena that change over time, such as stock prices, weather patterns, or disease outbreaks. By analyzing data over a prolonged period, researchers can identify patterns and trends that may not be apparent through cross-sectional research. Additionally, time series research can be used to evaluate the effectiveness of interventions or policies, allowing researchers to assess the impact of changes over time.
However, time series research also has its limitations. One of the primary drawbacks is the need for extensive resources, including large datasets and advanced statistical software. Additionally, time series research is susceptible to data quality issues, such as missing values or measurement errors, which can affect the validity and reliability of the findings. Furthermore, time series research can be prone to incorrect inference, particularly if the data is not properly adjusted for seasonality or trends.
In the context of the cross-sectional vs time series debate, time series research is often preferred when researchers need to examine trends and patterns over time. However, when researchers need to answer questions about the relationships between variables at a single point in time, cross-sectional research may be a more suitable approach. By understanding the advantages and limitations of time series research, researchers can make informed decisions about the most appropriate design for their study.
Ultimately, the choice between cross-sectional and time series research depends on the research question, data availability, and study goals. By considering the strengths and weaknesses of each approach, researchers can select the most appropriate design for their study, ensuring that their findings are valid, reliable, and generalizable.
How to Choose the Right Research Design for Your Study
Selecting the appropriate research design is a crucial step in ensuring the validity and reliability of a study’s findings. When it comes to choosing between cross-sectional and time series research, researchers must carefully consider their research questions, data availability, and study goals. In this section, we will provide guidance on how to make an informed decision about the most suitable research design for your study.
First and foremost, researchers should consider their research question. Is the study aimed at examining the relationships between variables at a single point in time, or is it focused on analyzing trends and patterns over time? If the former, cross-sectional research may be the more suitable approach. However, if the latter, time series research may be more appropriate.
Data availability is another critical factor to consider. Do you have access to a large dataset with multiple observations over time, or is your data limited to a single point in time? If you have access to longitudinal data, time series research may be a more suitable approach. However, if your data is limited to a single point in time, cross-sectional research may be more feasible.
Study goals are also an essential consideration. Are you looking to identify causal relationships between variables, or are you seeking to describe the characteristics of a population? If the former, time series research may be more suitable. However, if the latter, cross-sectional research may be more appropriate.
When weighing the pros and cons of each approach, researchers should consider the trade-offs between cost, time, and data quality. Cross-sectional research is often more cost-effective and easier to implement, but it may lack the longitudinal data needed to establish causal relationships. Time series research, on the other hand, may require more extensive resources and data quality issues, but it can provide valuable insights into trends and patterns over time.
Ultimately, the choice between cross-sectional and time series research depends on a careful consideration of the research question, data availability, and study goals. By understanding the strengths and limitations of each approach, researchers can make informed decisions about the most appropriate design for their study, ensuring that their findings are valid, reliable, and generalizable.
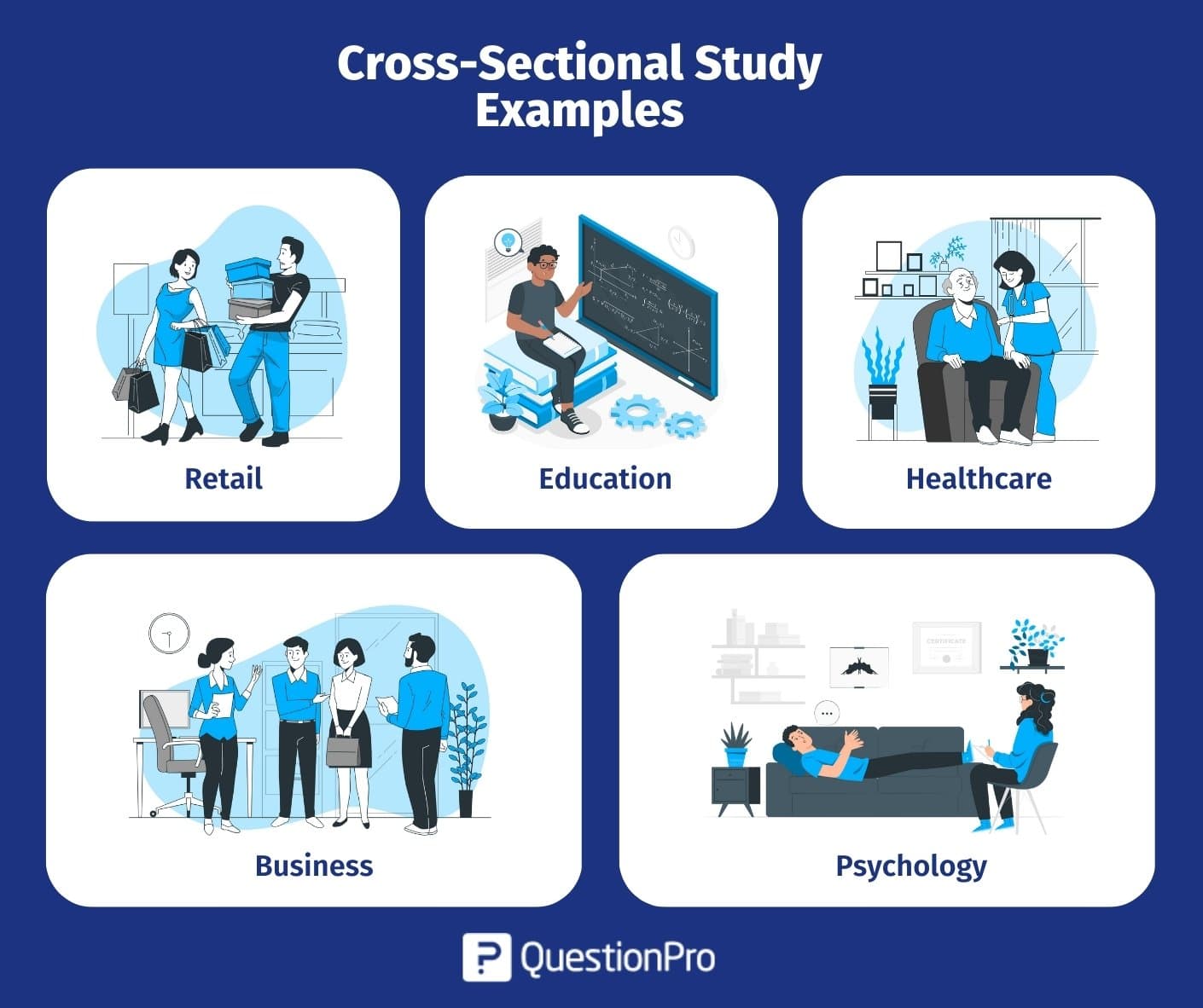
Real-World Applications of Cross-Sectional and Time Series Research
Cross-sectional and time series research designs have numerous practical applications across various fields, including economics, psychology, and healthcare. In this section, we will explore the strengths of each approach in different contexts, highlighting their real-world applications.
In economics, cross-sectional research is often used to study the relationships between economic variables, such as GDP and inflation rates, at a single point in time. For instance, a study examining the impact of trade policies on economic growth might employ a cross-sectional design to analyze data from multiple countries. On the other hand, time series research is commonly used to analyze trends and patterns in economic data over time, such as stock prices or unemployment rates.
In psychology, cross-sectional research is often used to study the characteristics of a population, such as personality traits or cognitive abilities, at a single point in time. For example, a study examining the relationship between personality traits and job performance might employ a cross-sectional design to analyze data from a sample of employees. Time series research, on the other hand, is commonly used to study the effects of interventions or treatments over time, such as the impact of cognitive-behavioral therapy on anxiety levels.
In healthcare, cross-sectional research is often used to study the prevalence of diseases or health outcomes at a single point in time. For instance, a study examining the relationship between smoking and lung cancer might employ a cross-sectional design to analyze data from a sample of patients. Time series research, on the other hand, is commonly used to study the trends and patterns of disease outbreaks or health outcomes over time, such as the spread of infectious diseases or the impact of vaccination programs.
In addition to these fields, cross-sectional and time series research designs have numerous applications in other areas, such as education, marketing, and environmental science. By understanding the strengths and limitations of each approach, researchers can select the most appropriate design for their study, ensuring that their findings are valid, reliable, and generalizable.
Ultimately, the choice between cross-sectional and time series research depends on the research question, data availability, and study goals. By recognizing the real-world applications of each approach, researchers can make informed decisions about the most suitable design for their study, leading to more effective and impactful research.
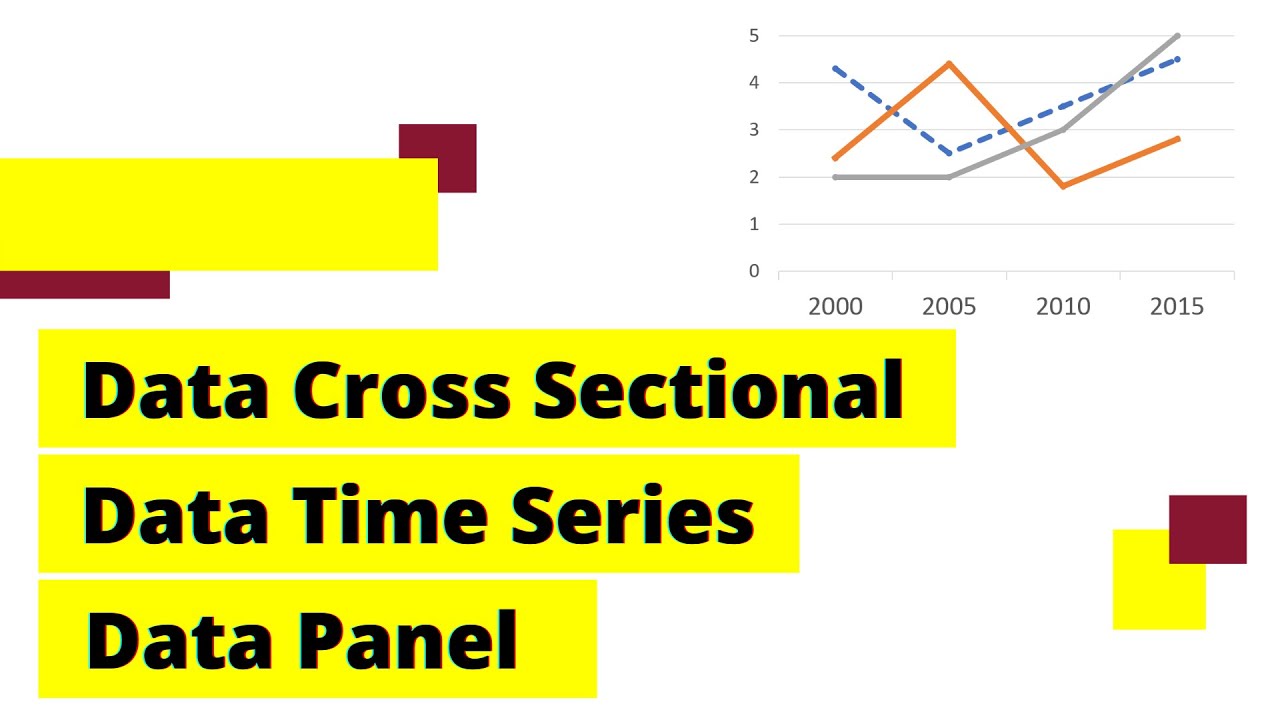
Common Pitfalls to Avoid in Cross-Sectional and Time Series Research
When conducting cross-sectional and time series research, it is essential to be aware of common pitfalls that can compromise the validity and reliability of the findings. In this section, we will discuss common mistakes researchers make when using these research designs, including sampling biases, data quality issues, and incorrect inference.
One common pitfall in cross-sectional research is sampling bias, which occurs when the sample is not representative of the population. This can lead to inaccurate conclusions and a lack of generalizability. For example, a study examining the relationship between education level and income may be biased if the sample only includes individuals from a specific socioeconomic background. To avoid this pitfall, researchers should ensure that their sample is diverse and representative of the population.
Data quality issues are another common pitfall in both cross-sectional and time series research. Poor data quality can lead to inaccurate conclusions and a lack of reliability. For example, a study examining the trends in stock prices over time may be compromised if the data is incomplete or inaccurate. To avoid this pitfall, researchers should ensure that their data is accurate, complete, and reliable.
Incorrect inference is another common pitfall in cross-sectional and time series research. This occurs when researchers draw conclusions that are not supported by the data. For example, a study examining the relationship between two variables may incorrectly infer causality when in fact the relationship is correlational. To avoid this pitfall, researchers should ensure that their conclusions are supported by the data and that they have controlled for potential confounding variables.
In time series research, another common pitfall is the failure to account for seasonality or trends in the data. This can lead to inaccurate conclusions and a lack of reliability. For example, a study examining the trends in sales over time may be compromised if it fails to account for seasonal fluctuations. To avoid this pitfall, researchers should ensure that they have accounted for seasonality and trends in the data.
By being aware of these common pitfalls, researchers can take steps to avoid them and ensure that their findings are valid, reliable, and generalizable. This includes ensuring that the sample is representative, the data is of high quality, and the conclusions are supported by the data. By doing so, researchers can increase the credibility and impact of their research.
In the context of cross-sectional vs time series research, it is essential to recognize the strengths and limitations of each approach and to avoid common pitfalls that can compromise the validity and reliability of the findings. By doing so, researchers can make informed decisions about the most suitable design for their study and increase the credibility and impact of their research.
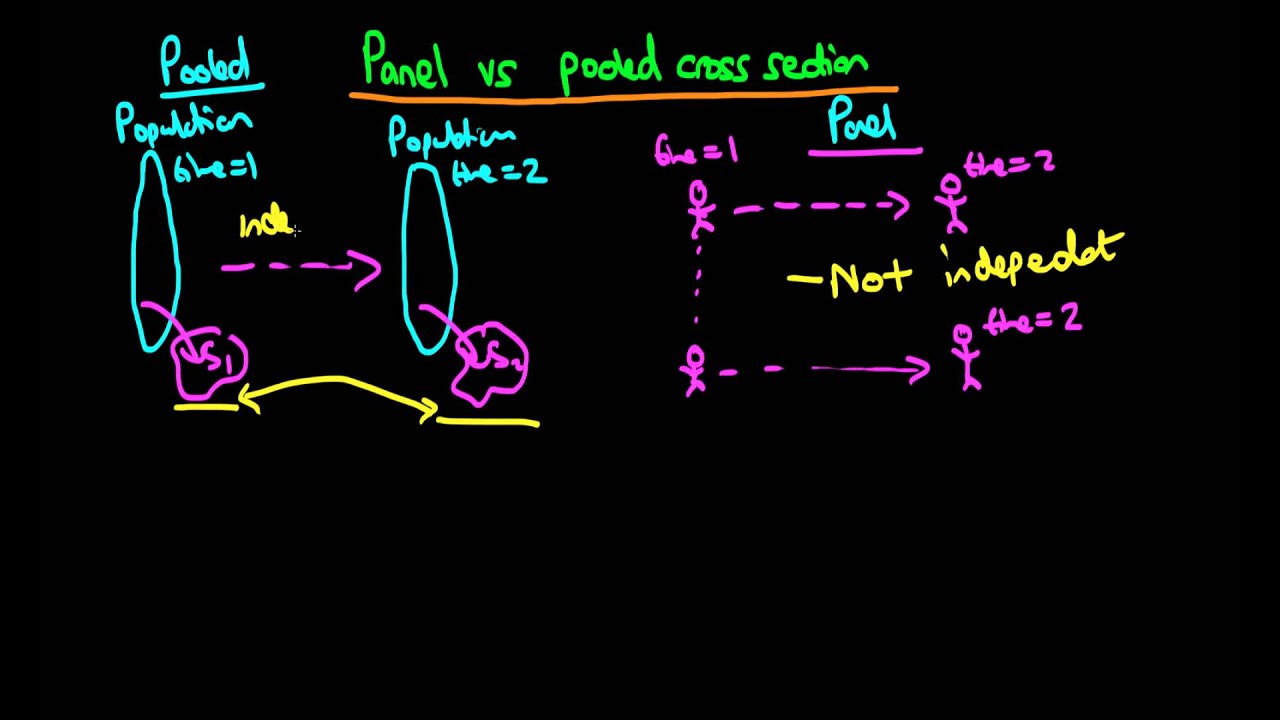
Best Practices for Combining Cross-Sectional and Time Series Research
Combining cross-sectional and time series research designs can offer a more comprehensive understanding of a research question, increasing the validity and generalizability of the findings. However, integrating both approaches requires careful consideration and planning. In this section, we will explore the benefits of combining cross-sectional and time series research and provide guidance on how to integrate both approaches effectively.
One of the primary benefits of combining cross-sectional and time series research is that it allows researchers to capture both the snapshot of a particular moment in time and the trends and patterns over time. This can provide a more nuanced understanding of the research question, enabling researchers to identify relationships and patterns that may not be apparent through a single approach. For example, a study examining the relationship between education level and income could use cross-sectional data to examine the current relationship and time series data to examine how this relationship has changed over time.
To combine cross-sectional and time series research effectively, researchers should ensure that the research questions and objectives are aligned with the strengths of each approach. This includes identifying the research questions that can be best answered through cross-sectional data and those that require time series data. Additionally, researchers should ensure that the data collection and analysis methods are compatible and that the data is of high quality.
Another key consideration when combining cross-sectional and time series research is the potential for data integration. This includes ensuring that the data is compatible and that the variables are measured consistently across both approaches. Researchers should also consider the potential for data fusion, where data from both approaches is combined to create a more comprehensive dataset.
In the context of cross-sectional vs time series research, combining both approaches can offer a more comprehensive understanding of the research question. By leveraging the strengths of each approach, researchers can increase the validity and generalizability of their findings, providing a more nuanced understanding of the research question. By following best practices for combining cross-sectional and time series research, researchers can ensure that their study is well-designed, well-executed, and provides valuable insights.
Ultimately, combining cross-sectional and time series research requires careful planning, consideration, and execution. By doing so, researchers can unlock the full potential of both approaches, providing a more comprehensive understanding of the research question and increasing the impact of their research.
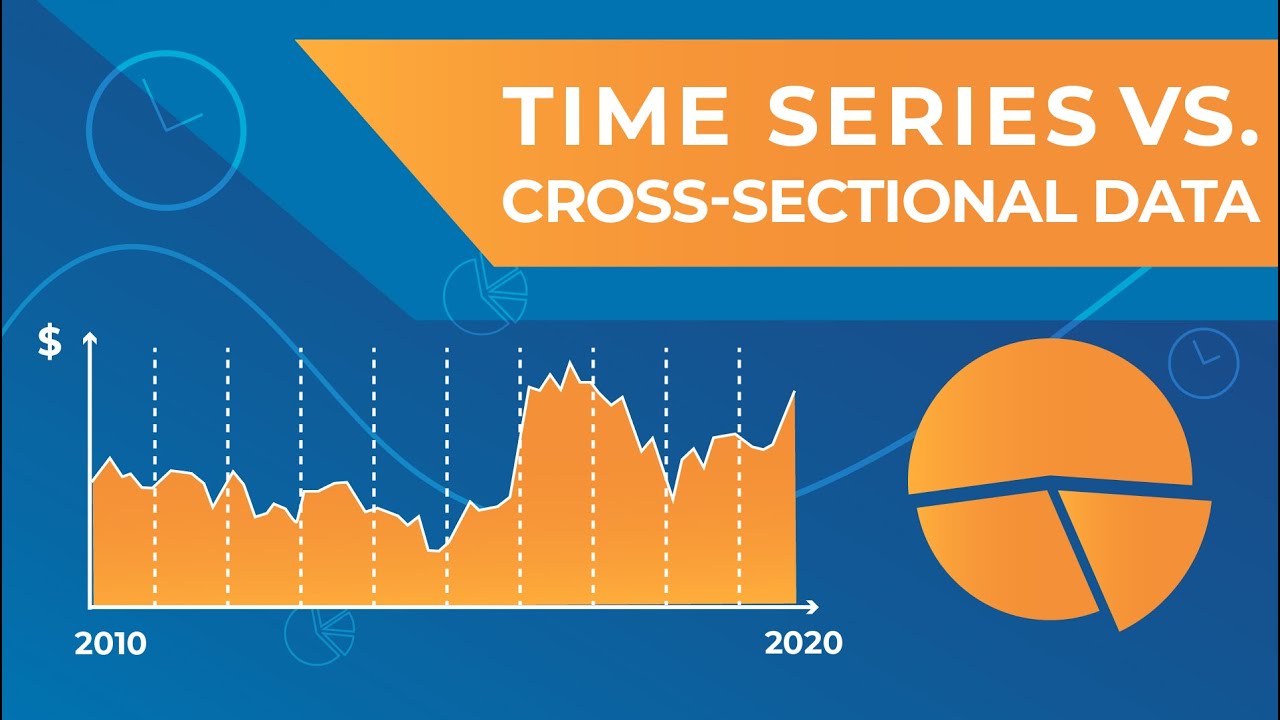
Future Directions in Research Design: Emerging Trends and Opportunities
The landscape of research design is constantly evolving, with new technologies and methodologies emerging to address the complexities of modern research questions. In the context of cross-sectional vs time series research, several emerging trends and opportunities are poised to revolutionize the field.
One of the most significant developments is the increasing use of machine learning and big data analytics in research design. These approaches enable researchers to analyze large datasets and identify patterns and relationships that may not be apparent through traditional methods. For example, machine learning algorithms can be used to analyze time series data and identify trends and patterns that can inform cross-sectional research.
Another emerging trend is the use of artificial intelligence (AI) in research design. AI can be used to automate data collection and analysis, freeing up researchers to focus on higher-level tasks such as data interpretation and theory development. Additionally, AI can be used to identify potential biases and errors in research design, increasing the validity and reliability of findings.
The increasing availability of large datasets and the development of new analytical tools are also opening up new opportunities for research design. For example, the use of sensor data and IoT devices is enabling researchers to collect high-frequency data on a wide range of phenomena, from environmental monitoring to human behavior. This data can be used to inform both cross-sectional and time series research, providing a more comprehensive understanding of complex systems.
In the context of cross-sectional vs time series research, these emerging trends and opportunities offer a range of benefits. By leveraging machine learning and big data analytics, researchers can increase the validity and generalizability of their findings, while also reducing the time and cost associated with data collection and analysis. Additionally, the use of AI and other emerging technologies can help to address common pitfalls in research design, such as sampling biases and data quality issues.
Ultimately, the future of research design is likely to be shaped by the intersection of emerging technologies and methodologies. By embracing these trends and opportunities, researchers can unlock new insights and understanding, and drive innovation in a wide range of fields.
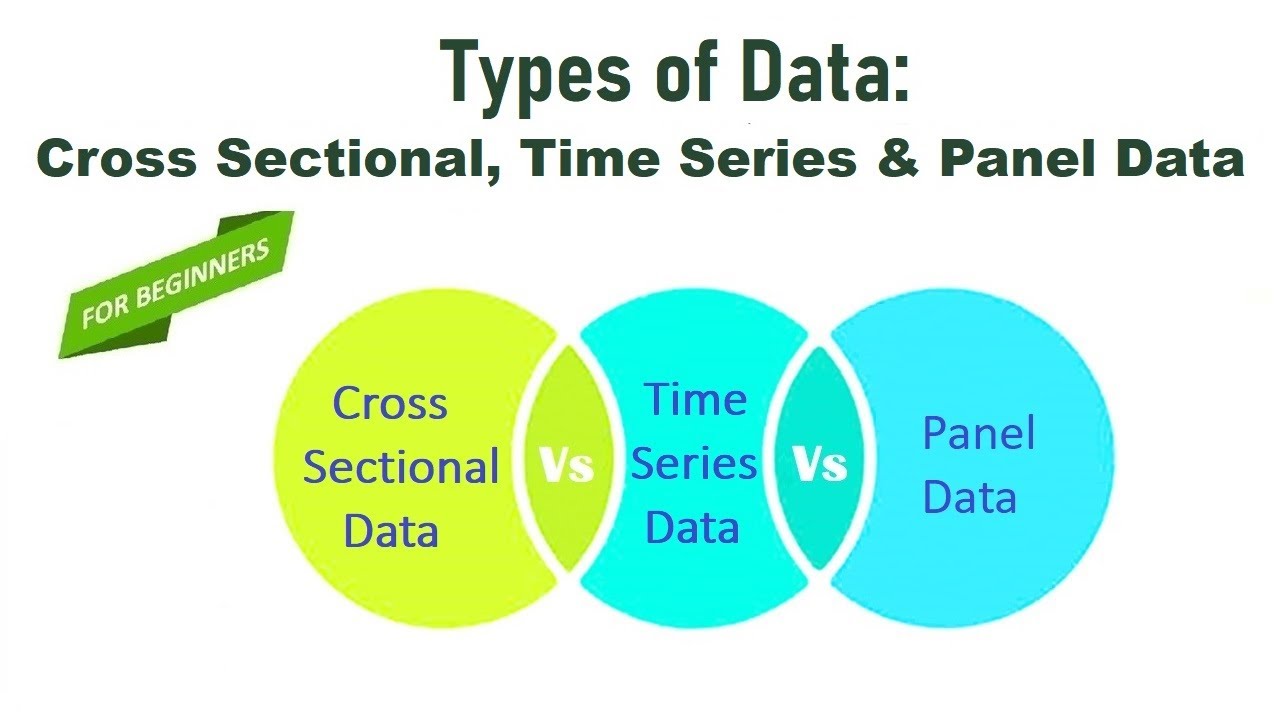