The Power of AI and Machine Learning in Trading
Artificial Intelligence (AI) and Machine Learning (ML) have revolutionized modern trading by enabling the analysis of vast amounts of data and making accurate predictions. These advanced technologies have opened up new possibilities for traders and investors, allowing them to make more informed decisions and optimize their trading strategies.
AI and ML can be applied in various aspects of trading, such as sentiment analysis, risk management, and automated trading systems. Sentiment analysis uses AI algorithms to interpret market sentiment by examining social media posts, news articles, and other public data sources. This information helps traders understand the overall attitude towards specific assets or the market in general, which can be valuable for predicting future price movements.
Risk management is another critical area where AI and ML excel. By analyzing historical data and market trends, these technologies can identify potential risks and suggest appropriate risk mitigation strategies. This proactive approach to risk management helps traders minimize losses and protect their investments in volatile markets.
Automated trading systems, also known as algorithmic trading, are another application of AI and ML in trading. These systems use predefined rules and complex algorithms to execute trades automatically, based on predefined criteria. Automated trading systems can significantly improve trading efficiency, reduce human error, and enable traders to capitalize on opportunities that might be too fast for human intervention.
By combining AI, ML, and quantum computing for trading, traders can unlock unprecedented accuracy, speed, and decision-making capabilities. However, it is essential to address the challenges and limitations associated with these advanced technologies, such as data privacy concerns, regulatory issues, and the need for specialized expertise.
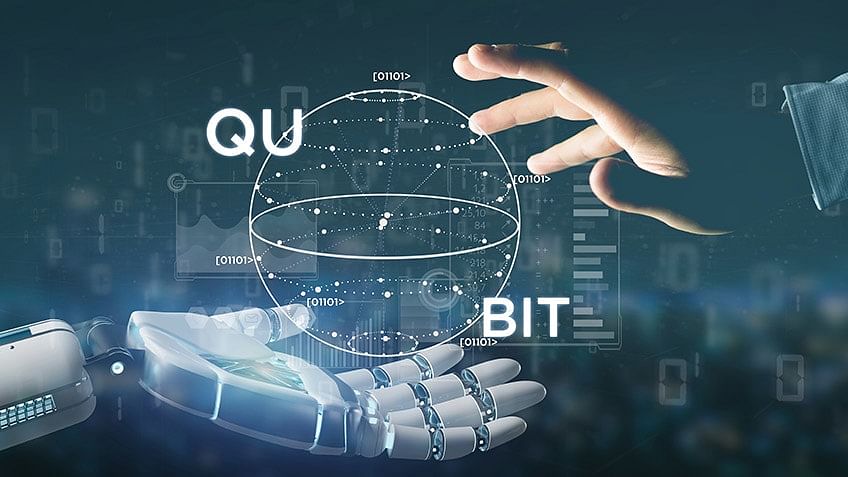
Exploring Quantum Computing in Trading
Quantum computing is an emerging technology that has the potential to revolutionize various industries, including trading. Unlike classical computers that use bits (0s and 1s) to process information, quantum computers use quantum bits, or qubits, which can exist in multiple states simultaneously. This unique property allows quantum computers to process complex calculations and simulations at unprecedented speeds.
In trading, quantum computing can enable real-time analysis of multiple scenarios, helping traders make more informed decisions and optimize their trading strategies. For instance, quantum computers can analyze vast amounts of data, such as historical price movements, market trends, and news articles, to predict future price movements with greater accuracy. This capability is particularly valuable in high-frequency trading, where split-second decisions can make a significant difference in profitability.
Moreover, quantum computing can improve risk management in trading by simulating various risk scenarios and suggesting appropriate risk mitigation strategies. By analyzing multiple variables and their potential impacts on a trader’s portfolio, quantum computers can help traders minimize losses and protect their investments in volatile markets.
However, integrating quantum computing into trading comes with its own set of challenges and limitations. For instance, quantum computers require specialized expertise to operate and maintain, and they are still in the early stages of development. Additionally, data privacy concerns and regulatory issues may arise as quantum computing becomes more prevalent in trading. Nevertheless, the potential benefits of quantum computing in trading, such as improved accuracy, faster processing times, and enhanced decision-making capabilities, make it an exciting area of research and development.
How to Combine AI, ML, and Quantum Computing for Trading
The integration of Artificial Intelligence (AI), Machine Learning (ML), and quantum computing for trading can lead to significant improvements in accuracy, processing times, and decision-making capabilities. By combining these technologies, traders can gain a competitive edge and optimize their trading strategies.
The first step in integrating AI, ML, and quantum computing for trading is to identify the specific use cases where this combination can provide the most significant benefits. For instance, traders can use AI and ML algorithms to analyze vast amounts of data and identify patterns and trends that can inform trading decisions. By incorporating quantum computing, traders can process this data at unprecedented speeds, enabling real-time analysis of multiple scenarios.
One potential application of this integration is in automated trading systems. By combining AI, ML, and quantum computing, traders can develop sophisticated algorithms that can analyze market data, identify trading opportunities, and execute trades automatically. These algorithms can take into account multiple variables and their potential impacts on a trader’s portfolio, enabling more informed and timely trading decisions.
Another application is in risk management. By simulating various risk scenarios and analyzing their potential impacts on a trader’s portfolio, AI, ML, and quantum computing can help traders minimize losses and protect their investments. This capability is particularly valuable in volatile markets, where the ability to analyze and respond to risk in real-time can make a significant difference in profitability.
However, integrating AI, ML, and quantum computing for trading also comes with its own set of challenges and limitations. For instance, the development and implementation of these technologies require specialized expertise and resources. Additionally, data privacy concerns and regulatory issues may arise as AI, ML, and quantum computing become more prevalent in trading. Nevertheless, the potential benefits of combining AI, ML, and quantum computing for trading, such as improved accuracy, faster processing times, and enhanced decision-making capabilities, make it an exciting area of research and development.
Challenges and Limitations of AI, ML, and Quantum Computing in Trading
While combining AI, ML, and quantum computing for trading offers numerous benefits, it also comes with its own set of challenges and limitations. One of the primary challenges is the need for specialized expertise. Developing and implementing AI, ML, and quantum computing algorithms requires a deep understanding of these technologies, as well as the financial markets. As a result, traders and organizations interested in implementing these technologies may face significant barriers to entry.
Data privacy is another concern when combining AI, ML, and quantum computing for trading. With the ability to process vast amounts of data, these technologies also raise questions about how that data is collected, stored, and used. Ensuring data privacy and security is essential to maintaining trust and compliance with regulations.
Regulatory issues are another challenge when combining AI, ML, and quantum computing for trading. As these technologies become more prevalent, regulators may need to develop new rules and guidelines to ensure fair and ethical practices. Staying informed about regulatory developments and ensuring compliance is essential for traders and organizations implementing these technologies.
Another limitation of combining AI, ML, and quantum computing for trading is the need for significant computational resources. Quantum computers, in particular, require specialized hardware and infrastructure, which can be expensive and challenging to maintain. Additionally, the accuracy and effectiveness of AI, ML, and quantum computing algorithms depend on the quality and availability of data, which can be a challenge in the financial markets.
Despite these challenges and limitations, the potential benefits of combining AI, ML, and quantum computing for trading make it an exciting area of research and development. By addressing these challenges and working to overcome these limitations, traders and organizations can unlock the full potential of these technologies and gain a competitive edge in the financial markets.
Real-World Applications of AI, ML, and Quantum Computing in Trading
Combining AI, ML, and quantum computing for trading is no longer a theoretical concept, but a reality for many organizations. Several companies and projects have successfully implemented these technologies to enhance their trading strategies and gain a competitive edge.
One example is the use of AI and ML algorithms for sentiment analysis in trading. By analyzing social media posts, news articles, and other forms of unstructured data, these algorithms can identify trends and patterns that may indicate changes in market sentiment. This information can then be used to inform trading decisions, enabling traders to react more quickly to market movements.
Risk management is another area where AI and ML are being used in trading. By analyzing historical data and identifying patterns, these algorithms can help traders to better understand and manage risk. For example, ML algorithms can be used to identify potential risks in a trader’s portfolio and suggest alternative investments to mitigate those risks.
Automated trading systems are another application of AI and ML in trading. By using algorithms to analyze market data and execute trades automatically, these systems can help traders to make faster and more informed decisions. Additionally, automated trading systems can help to reduce the impact of human emotions on trading decisions, leading to more consistent and profitable outcomes.
Quantum computing is also being explored for its potential impact on trading. By enabling real-time analysis of multiple scenarios, quantum computers can help traders to make more informed decisions and react more quickly to market movements. For example, quantum computers can be used to analyze complex financial instruments, such as derivatives, and identify potential risks and opportunities.
One project that is exploring the use of quantum computing in trading is the IBM Q Network. This network brings together researchers, developers, and traders to explore the potential of quantum computing in finance. By providing access to quantum computers and expertise, the IBM Q Network is helping to accelerate the development and implementation of quantum computing in trading.
Another example is the use of quantum computing for portfolio optimization. By analyzing large datasets and identifying patterns, quantum computers can help traders to optimize their portfolios and maximize returns. For example, a quantum computer can be used to analyze a trader’s portfolio and suggest alternative investments to improve diversification and reduce risk.
In conclusion, combining AI, ML, and quantum computing for trading offers numerous benefits, including improved accuracy, faster processing times, and enhanced decision-making capabilities. While there are challenges and limitations to implementing these technologies, several companies and projects have successfully implemented them to enhance their trading strategies and gain a competitive edge. As these technologies continue to evolve and improve, we can expect to see even more innovative applications in the world of trading.
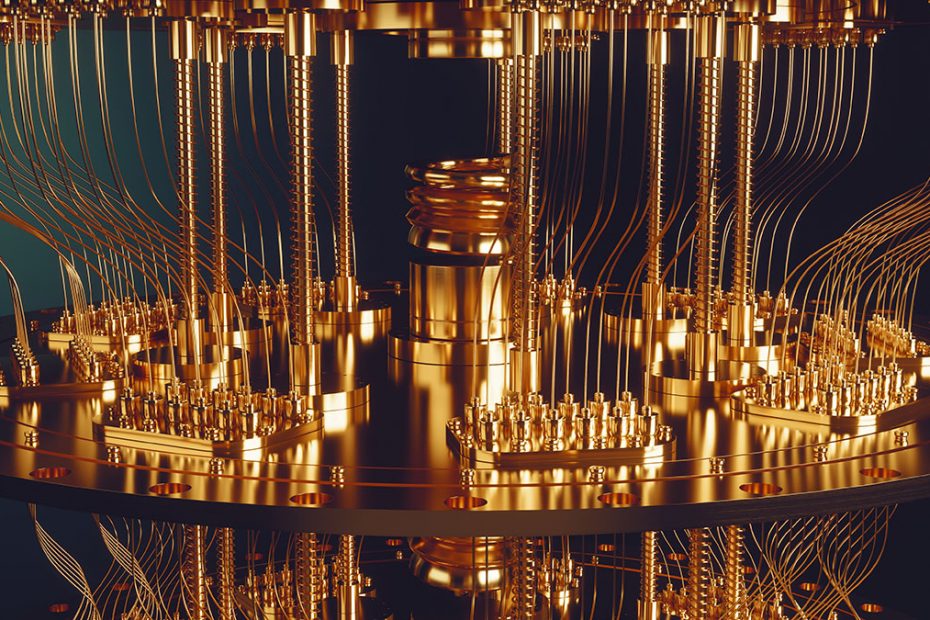
Future Perspectives: AI, ML, and Quantum Computing in Trading
As we look to the future of trading, it is clear that combining AI, ML, and quantum computing will play an increasingly important role. These technologies offer numerous benefits, including improved accuracy, faster processing times, and enhanced decision-making capabilities. However, there are also challenges and limitations that must be addressed in order to fully realize their potential.
One emerging trend in the field of AI and ML in trading is the use of deep learning algorithms. These algorithms, which are modeled after the structure and function of the human brain, are capable of analyzing vast amounts of data and identifying complex patterns and relationships. By using deep learning algorithms in trading, traders can gain a deeper understanding of market dynamics and make more informed decisions.
Another trend is the use of natural language processing (NLP) algorithms in trading. NLP algorithms are capable of analyzing and interpreting human language, enabling traders to use unstructured data, such as news articles and social media posts, to inform their trading decisions. By using NLP algorithms in trading, traders can gain a more complete picture of market sentiment and identify potential opportunities and risks.
Quantum computing is also expected to have a significant impact on the future of trading. As quantum computers become more powerful and accessible, they will enable traders to perform complex calculations and simulations at unprecedented speeds. This will enable real-time analysis of multiple scenarios, enabling traders to make more informed decisions and react more quickly to market movements.
However, there are also challenges and limitations to using AI, ML, and quantum computing in trading. One of the main challenges is data privacy. As these technologies rely on large amounts of data, there is a risk that sensitive information could be compromised. Additionally, there are regulatory issues to consider, as the use of AI, ML, and quantum computing in trading is still largely uncharted territory. Finally, there is a need for specialized expertise in order to effectively implement and use these technologies.
Despite these challenges, the potential benefits of combining AI, ML, and quantum computing for trading are significant. As these technologies continue to evolve and improve, we can expect to see even more innovative applications in the world of trading. It is important for traders and organizations to stay informed about these developments and consider how they can leverage these technologies to gain a competitive edge.

Getting Started with AI, ML, and Quantum Computing in Trading
For traders and organizations interested in implementing AI, ML, and quantum computing in their trading strategies, there are several steps to consider. First, it is important to have a clear understanding of the technology and its potential applications in trading. This can be achieved through research, attending industry events, and networking with experts in the field.
Next, it is important to assess the organization’s current capabilities and infrastructure. This includes evaluating the availability of data, the existing technology stack, and the level of expertise within the organization. Based on this assessment, a plan can be developed for integrating AI, ML, and quantum computing into the trading strategy. This plan should include specific goals, timelines, and resources required.
There are also several resources available to help traders and organizations get started with AI, ML, and quantum computing in trading. These include online courses, tutorials, and forums, as well as consulting services and technology providers that specialize in these areas. It is important to carefully evaluate these resources and choose those that align with the organization’s goals and capabilities.
When implementing AI, ML, and quantum computing in trading, it is important to follow best practices to ensure success. This includes ensuring data privacy and security, complying with relevant regulations, and maintaining transparency in the decision-making process. Additionally, it is important to continuously monitor and evaluate the performance of the technology and make adjustments as needed.
In conclusion, combining AI, ML, and quantum computing for trading offers numerous opportunities for traders and organizations. By following the steps outlined above, traders and organizations can successfully implement these technologies and gain a competitive edge in the market. It is important to stay informed about the latest developments in this rapidly evolving field and consider the ethical implications of using these technologies in trading.
Conclusion: The Unprecedented Opportunities of AI, ML, and Quantum Computing in Trading
Combining Artificial Intelligence (AI), Machine Learning (ML), and Quantum Computing for trading is a rapidly evolving field that offers numerous opportunities for traders and organizations. By harnessing the power of these technologies, traders can gain a competitive edge in the market through improved accuracy, faster processing times, and enhanced decision-making capabilities.
The integration of AI and ML in trading has already shown promising results, with applications such as sentiment analysis, risk management, and automated trading systems. These technologies have the ability to analyze vast amounts of data and make accurate predictions, enabling traders to make informed decisions and optimize their trading strategies.
Quantum computing, on the other hand, has the potential to revolutionize trading by processing complex calculations and simulations at unprecedented speeds. This can enable real-time analysis of multiple scenarios, allowing traders to react quickly to changing market conditions and capitalize on opportunities as they arise.
However, it is important to note that combining AI, ML, and quantum computing for trading also presents challenges and limitations. These include data privacy concerns, regulatory issues, and the need for specialized expertise. It is crucial for traders and organizations to carefully consider these factors and implement best practices to ensure the responsible use of these technologies.
Despite these challenges, the potential benefits of combining AI, ML, and quantum computing for trading are significant. Companies and projects such as IBM, Google, and Goldman Sachs have already begun to explore the possibilities of this technology, with promising results. These success stories illustrate the potential of combining AI, ML, and quantum computing for trading and serve as a testament to the power of these technologies.
In conclusion, combining AI, ML, and quantum computing for trading is a rapidly evolving field that offers unprecedented opportunities for traders and organizations. By staying informed about the latest developments and best practices, traders can harness the power of these technologies to gain a competitive edge in the market and achieve long-term success.
